Optimize AI Model Performance with MLOps
Enhance your AI model lifecycle with end-to-end automation and advanced insights, keeping you at the forefront of the AI revolution.
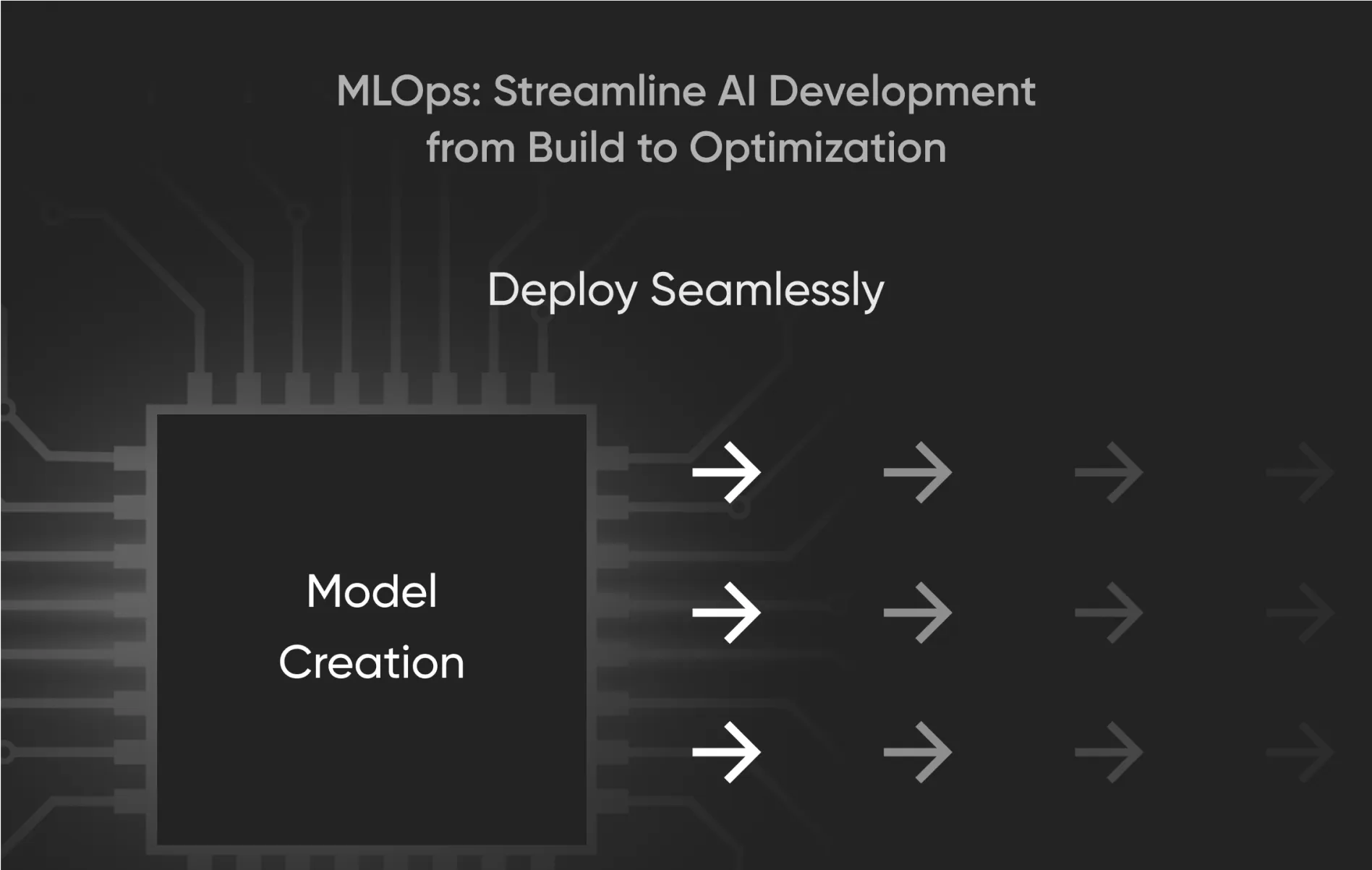
Accelerate AI Model Development with MLOps
Stay ahead in the AI ecosystem by streamlining your model creation, infrastructure monitoring, and cost management. Our MLOps solutions help you achieve high-performance AI models while maintaining cost efficiency and operational visibility.
End-to-End Automation
Streamline every phase of AI model development, from data processing to deployment, ensuring faster go-to-market and fewer manual interventions
Real-Time Infrastructure Monitoring
Gain full visibility into AI performance and resource usage, allowing for proactive adjustments and preventing costly downtimes or inefficiencies.
Cost-Effective Scaling
Optimize infrastructure usage with advanced cost-reduction techniques, ensuring your AI models scale efficiently without exceeding budget constraints.
Industries We Empower
with AI Solutions
Transform your business with tailored AI solutions across various sectors, improving operational efficiency, reducing costs, and scaling effortlessly.
Our AI MLOps Platform
in Action

Performance
Insights
Gain deep performance insights by monitoring and optimizing AI models in real time with MLOps.
Optimized for
Efficiency
Optimize for efficiency by streamlining model deployment, monitoring, and updates with MLOps.
Scalability on Demand
Scale effortlessly on demand, expanding AI capabilities as your needs grow.
Continuous Model
Improvement
Ensure continuous model improvement with automated monitoring, retraining, and performance optimization.
Trusted by Leading Enterprises: Hear What Our Clients Have to Say









